In the evolving landscape of artificial intelligence (AI) and machine learning (ML), neural networks are at the forefront of technological advancements. One such fascinating model is the cellular neural network (CNN), which brings a unique structure and operation style that differentiates it from traditional neural networks. As industries demand faster processing capabilities and real-time solutions, understanding the framework and benefits of cellular neural networks becomes increasingly important. This blog aims to provide a comprehensive overview of cellular neural networks, including their concept, history, types, applications, advantages, and future prospects.
Definition and Concept of Cellular Neural Networks
A cellular neural network is a form of parallel computing architecture where the structure consists of an array of identical processing units, also called cells. Each cell in a cellular neural network interacts only with its neighboring cells, allowing for localized processing of information. The main difference between CNNs and traditional neural networks is this locality feature, making Cellular Computing Networks more suited for tasks that require high-speed, real-time processing with minimal global communication.
Basic Principles and Structure
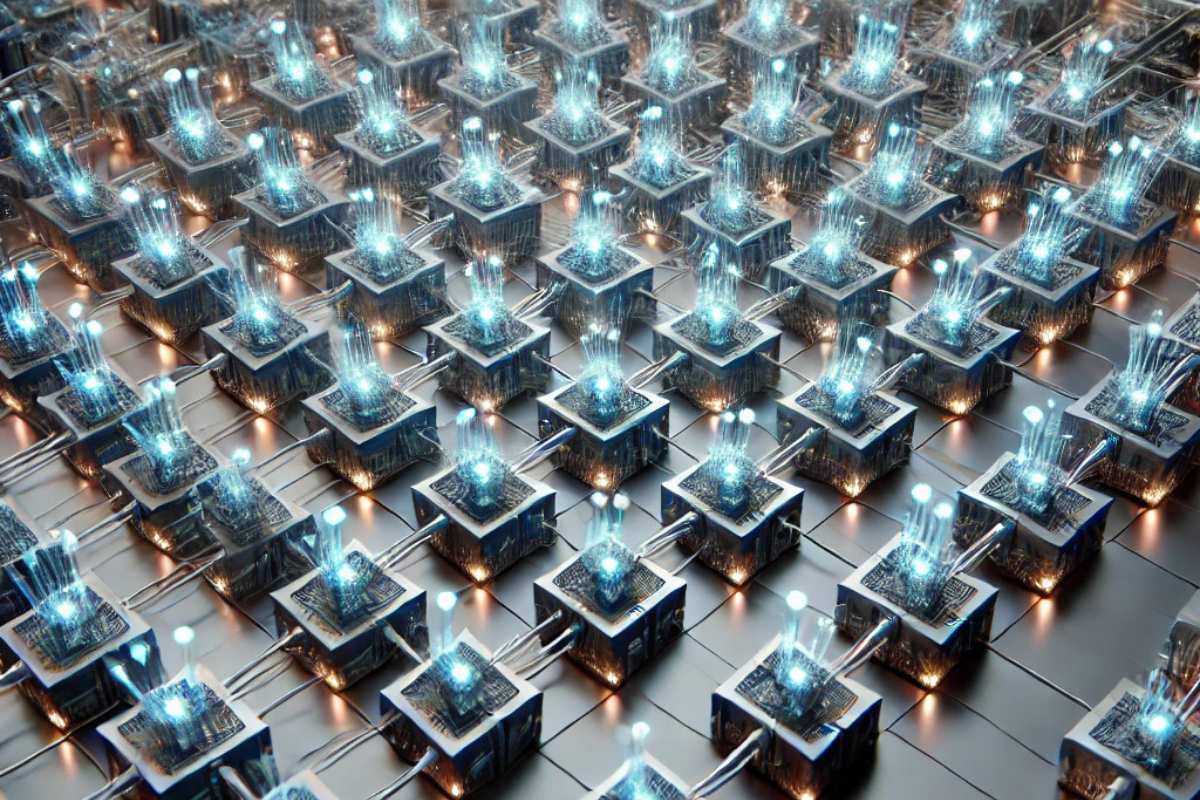
The basic structure of a cellular neural network consists of a grid of cells, where each cell has its own processing unit. These cells are connected to their nearest neighbors and operate simultaneously in parallel. The connectivity of these cells to their immediate surroundings is the hallmark of a cellular neural network. Each cell follows a set of predefined rules, which dictate how it interacts with its neighboring cells. These interactions enable CNNs to handle tasks like image processing and pattern recognition efficiently.
Comparison with Traditional Neural Networks
Unlike traditional neural networks, which rely on full connectivity between layers, Cellular Computing Networks are characterized by local interactions, which result in a distributed processing system. This localized processing leads to faster computational times, as each cell only processes information from its immediate neighbors. While traditional neural networks are better suited for tasks requiring global communication and learning, Cellular Computing Networks excel in tasks that require parallel, localized processing, like real-time image and signal processing.
Historical Background
The concept of Cellular Computing Networks was first introduced in 1988 by Leon Chua and Lin Yang. These researchers laid the foundation for CNNs by describing them as an extension of cellular automata with neural network-like processing capabilities. Their work aimed to develop a system that could perform computations quickly using localized data exchange, much like how biological systems operate.
Types of CNNs
Cellular Computing Networks come in various types, each tailored to specific use cases and processing needs. Below are the major types of CNNs:
- Standard Cellular Computing Networks (CNNs)
These are the original models introduced by Chua and Yang. Standard CNNs consist of cells that operate on continuous signals and are best suited for tasks like image processing and signal filtering. Each cell in a standard CNN has its own input, output, and processing function, which interacts with its neighbors.
- Variants and Extensions
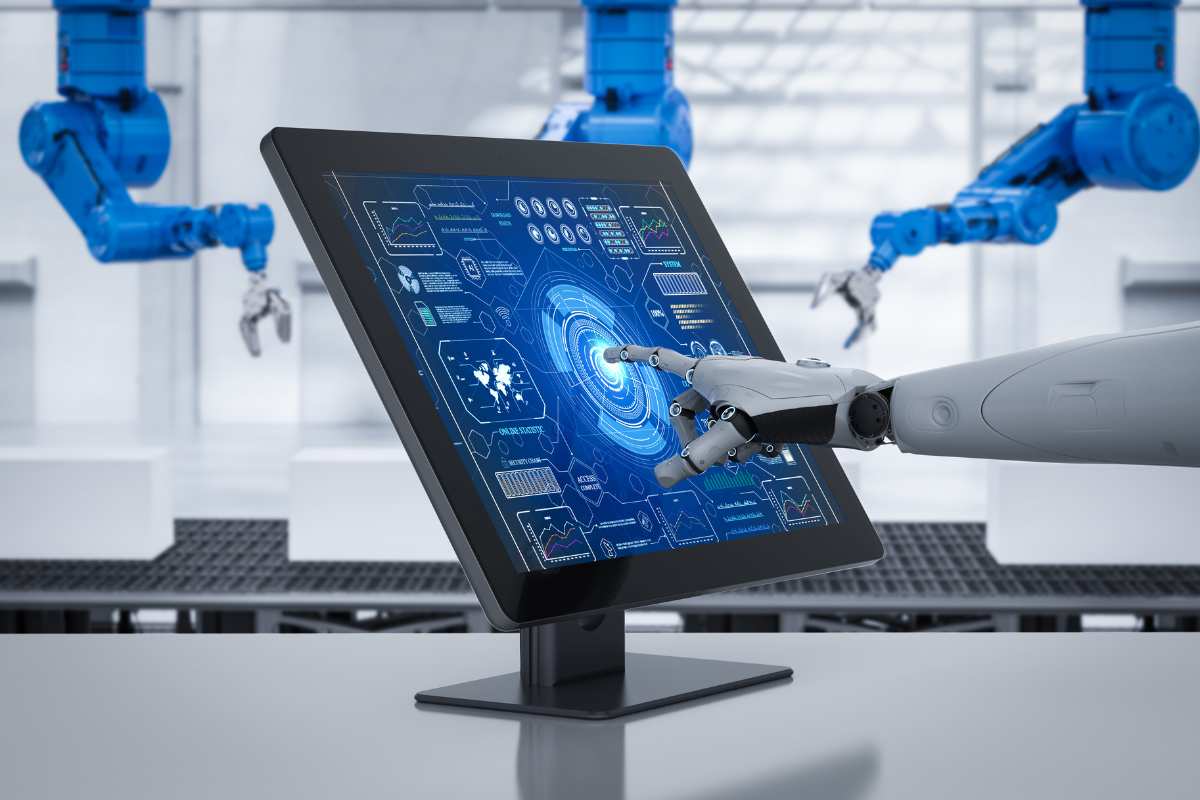
Several variants of Cellular Computing Networks have been developed to handle more specific tasks. These include discrete Cellular Computing Networks, where signals are processed in discrete time steps rather than continuously. Additionally, hybrid models have been developed to combine features of both discrete and continuous CNNs, allowing for greater flexibility in tasks like control systems and robotics.
Applications of Cellular Computing Networks
The diverse architecture of Cellular Computing Networks lends itself to a wide range of applications. Their ability to process information in parallel and in real-time makes them valuable in fields requiring high-speed computations and localized data processing.
1. Image and Signal Processing
One of the most prominent applications of CNNs is in image and signal processing. Cellular Computing Networks can efficiently handle tasks like edge detection, noise reduction, and pattern recognition due to their local processing capabilities. In real-time video processing, CNNs are used to filter frames, making them invaluable in surveillance and robotics.
2. Control Systems and Robotics
In control systems, Cellular Computing Networks offer a distributed approach to managing dynamic environments. Robots equipped with CNN-based control systems can process sensory inputs in real time and make decisions based on local information. This feature makes CNNs suitable for tasks like autonomous navigation and industrial automation.
3. Biomedical Applications
Cellular Neural Networks have found applications in biomedical engineering, where they are used for tasks like medical image analysis and signal filtering. For instance, Cellular Computing Networks are employed to detect patterns in medical imaging, such as identifying tumors in MRI scans. Their real-time processing capabilities allow for faster diagnostics and more efficient analysis of complex biomedical data.
Advantages and Disadvantages
Like any technological tool, Cellular Computing Networks have their own advantages and challenges.
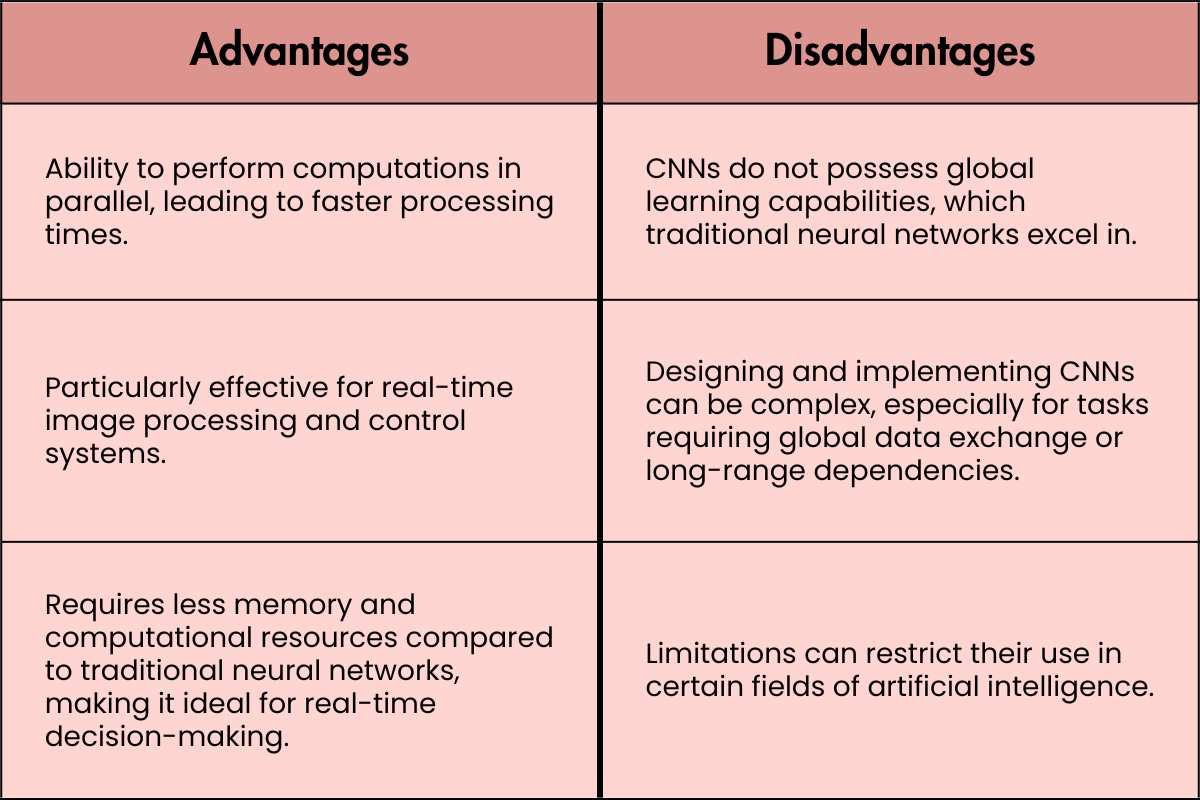
Future Prospects
The future of Cellular Computing Networks is promising, with potential advancements in both their architecture and applications. As industries continue to demand faster and more efficient real-time processing systems, CNNs are likely to play a larger role in fields like autonomous driving, robotics, and AI-based healthcare solutions.
Conclusion
Cellular neural networks offer a unique and powerful approach to parallel, real-time computation. With their roots in the 1980s and a bright future ahead, CNNs have proven themselves invaluable in fields like image processing, robotics, and biomedical engineering. While they come with certain limitations, the potential for growth and evolution makes them a crucial component of the broader artificial intelligence landscape. As technology advances, CNN are poised to play an increasingly important role in shaping the future of intelligent systems.
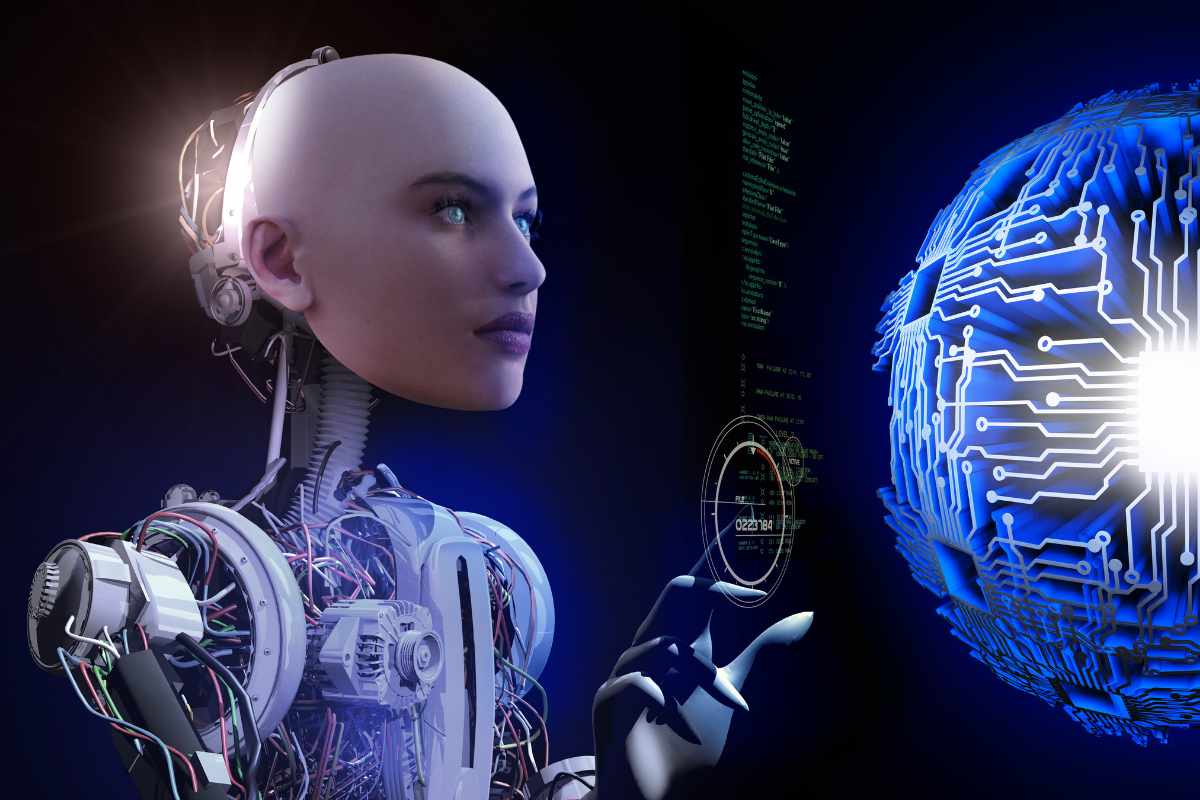
Artificial Intelligence Imaging: Transforming the Future of Medical Diagnostics
Artificial intelligence has transformed the face of many industries, but its entry into healthcare, particularly to medical imaging, has changed diagnostics and treatment planning.